Schwab's Thematic Research Approach
An innovative way to invest in our fast-changing world
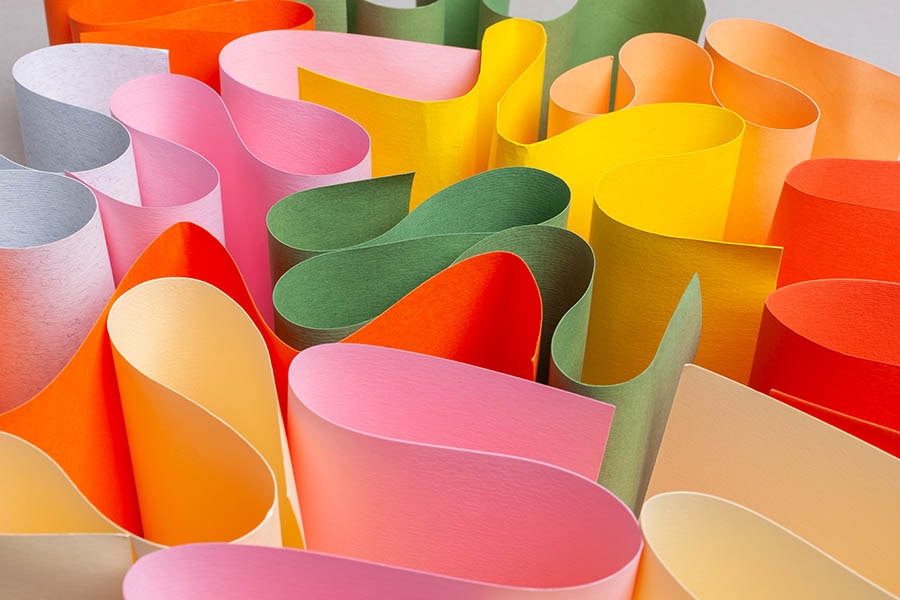
Consider the changes capturing investors' attention today: a rapid digital transition has pushed more consumers online; clean energy efforts have gained traction around the world; and artificial intelligence has changed the pace of innovation. The list goes on, from the growth of emerging markets such as China to increased spending on household pets. In each case, these areas could represent transformational trends with long-term potential that may be of interest to investors in the coming years.
In recent years, the world has experienced an explosion of information. The ability to collect and analyze data has changed how consumers shop, how companies make and sell products, and even how we treat illness and disease.
It has also changed how we invest. These days, it's easier for investors to access huge volumes of digital documents issued by publicly traded companies, including annual and quarterly earnings updates, revenue reports, and other financial metrics. Meanwhile, companies may have a wide variety of business lines that impact their bottom line and offer potential opportunities to investors. Investors can try and apply this information to better understand what may be driving that company's growth potential. At the same time, data can help investors connect a company to a particular investment theme. Thematic investing offers investors a way of categorizing companies not by sector or size but by the exposure they may offer to these trends.
The key, of course, is not to just collect useful data but to analyze it in a way that gives investors relevant information to help drive their investment decisions. At Schwab, our thematic research employs a unique approach to find, select, and measure stocks based on their relevance to a theme. To do this, we use a proprietary algorithm to help measure thematic relevance. This systematic approach helps ensure the stock is selected for its thematic relevance instead of past price performance or fundamental metrics such as revenue or earnings growth. In this way, our thematic stock research differs from other types of stock research, including Schwab's other proprietary stock research called Schwab Equity Ratings®.
Our algorithm is powered by natural language processing (NLP), which gives computers the power to read, analyze, and determine the significance of human language. We call this application of NLP "the Model," and it serves as the cornerstone of our modern approach to thematic research. The Model's capabilities allow the algorithm to sort through millions of documents to identify and rank companies based on their relevance to a particular investment theme. For example, when applying the Model to company annual reports, the NLP Model can digest thousands of pages of text and quantify thematic relevance for companies in seconds. In short, a task that might take days for a human to accomplish can be done in a fraction of the time.
As a result, the Model offers a way to help identify companies that have different levels of exposure to different investment themes. It also is designed to create thematic stock lists that can be regularly updated to help stay on the leading edge of a shifting investing environment. Investors should always consider each list of stocks in the context of their overall investment strategy. That includes weighing their objectives, risk tolerance, financial situation, and other individual factors to help ensure this thematic approach aligns with their unique strategy and goals.
Inside the Thematic Investing Process
Step 1: Identify and Define Theme
The first step is to identify and define a particular theme and its scope. Schwab's thematic research team begins this process by searching for investable opportunities across different sectors of the economy, such as consumer retail, technology, health care, energy, finance, and real estate. A theme might be tightly focused, providing exposure to a specific concept such as cloud computing. Or it could take a broader view of the shifting trends that are shaping our global economy, such as an aging population.
Step 2: Select Data Sources
A list of keywords and data sources are selected for the Model to use in its analysis. These data sources can include:
- Regulatory Filings: Companies that trade in the U.S. are required to make certain disclosures to regulators. These registration statements, periodic reports, and other forms must be filed electronically and are available to be retrieved and analyzed. These filings may offer insights into a company's areas of investment, emerging R&D efforts, or possible plans to move into new markets or competitive arenas.
- Patent Grants: When a company wants to claim exclusive use in the U.S. for an invention, they apply with the U.S. Patent and Trademark Office (USPTO). If the USPTO grants the patent, documents are created that can contain a wealth of details about the innovation—and may even offer clues on the company's future growth prospects.
- Clinical Trials: Before offering a drug or therapy to the public, a company often must conduct clinical trials to prove the drug or therapy's effectiveness and safety. In the U.S., these companies must submit the results of the trials to a public government database. Clinical trial filings may offer information on how far along a company is in the sometimes years-long drug development process or suggest the potential addressable market for a new pharmaceutical treatment.
Step 3: Calculate Theme Relevance
In this third step, the NLP advanced search algorithm searches these datasets, looking for specific keywords and determining how relevant each document is to the theme. By linking the documents back to the company that filed them, the Model can then more accurately identify companies that are relevant to the theme.
Since some companies will be more relevant to the theme than others, the Model assigns each one a theme relevance score. Take corporate filings: The Model's algorithm assigns higher scores to documents that mention a keyword or phrase more frequently. And when using multiple search phrases, a phrase that is common across many documents has a smaller contribution to the overall score than one that is relatively rare. The algorithm not only gives insight into how many times a phrase appears but also its relevancy to the document and the wider universe of source material.
Timing also matters: The algorithm considers documents and filings recent enough to still be relevant. Size is important, too. Larger companies may have more documents or data points that are relevant to a particular theme. But that doesn't mean they represent better opportunities for investors. That's why scores are systematically adjusted across several factors to offer an appropriate comparison of large and small companies' relevance to a theme.
Step 4: Construct the Stock List
Once the Model identifies the U.S. listed stocks and American Depositary Receipts (ADRs) and removes any with a zero-theme relevance score, the next step is to use the relevancy scores to build a list of securities with exposure to a theme. Securities that don't meet minimum market capitalization and liquidity levels are filtered out to remove the smallest micro-cap companies and illiquid stocks. For companies with multiple share classes, the share class that is most liquid to represent the company is selected.
Once these filters are applied, the companies are systematically ranked by their theme relevance score, and up to the top 25 stocks are selected. The result is a list of stocks that are relevant to a particular theme.
This list of stocks then has initial weights assigned to each stock based on a combination of theme relevance and market capitalization relative to the other stocks in the theme. Typically, stocks with the most theme relevance and higher market cap will have a higher percent weight in the theme. The total of the stock weights within the theme always equals 100%.
The weight of each stock changes over time based on the stock price changes.
We rerun the Model periodically to update the stock list for each theme and the weight of each stock within each theme. During this process, the Model reviews all the qualitative inputs, such as keywords, phrases, and sources that are searched. The aim is to continue to track themes as they evolve while also reducing turnover.
Looking Toward the Future
In today's highly competitive market environment, industries and companies are constantly seeking new avenues of growth. That may mean capitalizing on unique and disruptive innovations or on shifts in demographics or consumer behaviors that may open new market opportunities. Upstart companies that have reimagined business as usual may rise to become leaders in their industries. And emerging trends may make certain industries more relevant than ever or present well-positioned companies with potential opportunities to expand and grow. Our research focuses on trends that have the potential to be transformational and long term—not on short-term trends that are here today and may be gone tomorrow.
Disruption and change can also bring about opportunity. For investors, these opportunities can be challenging to uncover. While they may identify trends that they believe offer growth potential, finding the individual investments that allow them to take advantage of those growth prospects can be a time-consuming and labor-intensive process. With our approach to thematic investing, investors can more efficiently invest in what they believe in, helping them in the search for growth potential in today's—and tomorrow's—market.